Foot Traffic
Definition
Foot Traffic is a collection of metrics providing additional context to what is happening at a given location. Visits estimates the amount of unique people with a stay at a location in a day. Any person is included in this count, including workers and residents. The monthly data is produced on calendar months. New data is made available by the 5th of each month. The weekly data is produced on Sunday to Monday weeks. New data is made available on Wednesdays.
The metrics is reported in the following values:
visits_sum
: the sum of daily visits across the aggregation period (week and month)visits_p50
: the typical (median) daily visits across the aggregation period (week and month)
The Visit metric estimates are derived from Unacast's proprietary machine learning model.
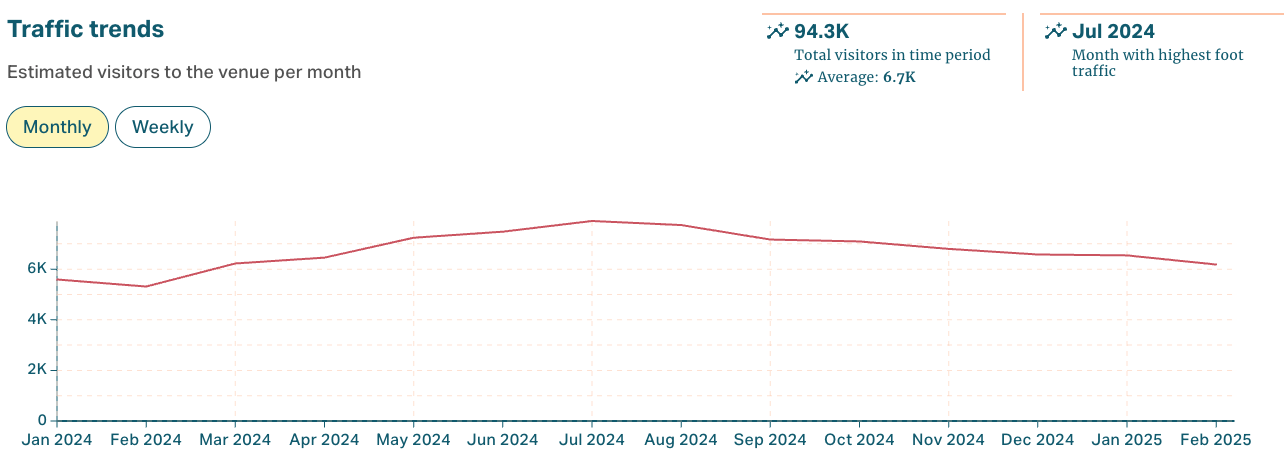
What questions does it answer?
- How do visits to a specific location change over time?
- How do visit patterns differ between locations?
- How does visitation at key locations change before, during, and after a specific event?
- What is the seasonality of visits to a location?
- Which locations are experiencing the highest visitation growth or decline?
- What proportion of total foot traffic does each location account for in a region?
- Are there any correlations between foot traffic and other datasets?
Methodology
We estimate the visits to a location by using a machine learning model. The model learns the relationships between various types of context and person count of that location. One important input to our model is derived from privacy-friendly 1st party GPS data. We aggregate that data on the 1st party side and use the derived aggregates on a hexagon level as input to our ML model.
Unlike typical aggregated products that rely solely on aggregating the underlying GPS device-level supply, our machine learning model is more robust and less dependent on GPS data fluctuations because it is based on a magnitude of different data sources to estimate visits.
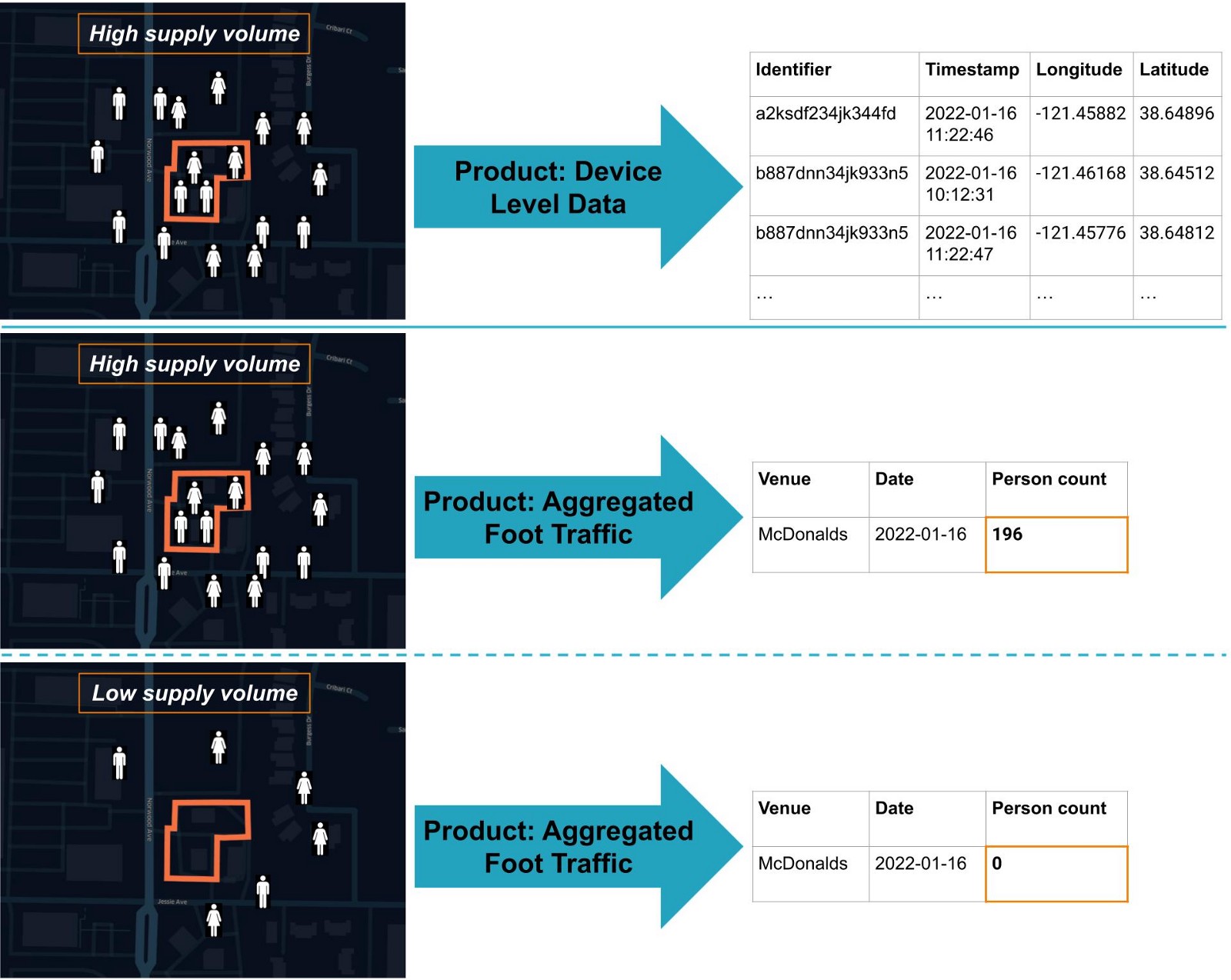
Using machine learning, we are able to overcome these supply problems and create a more robust product.
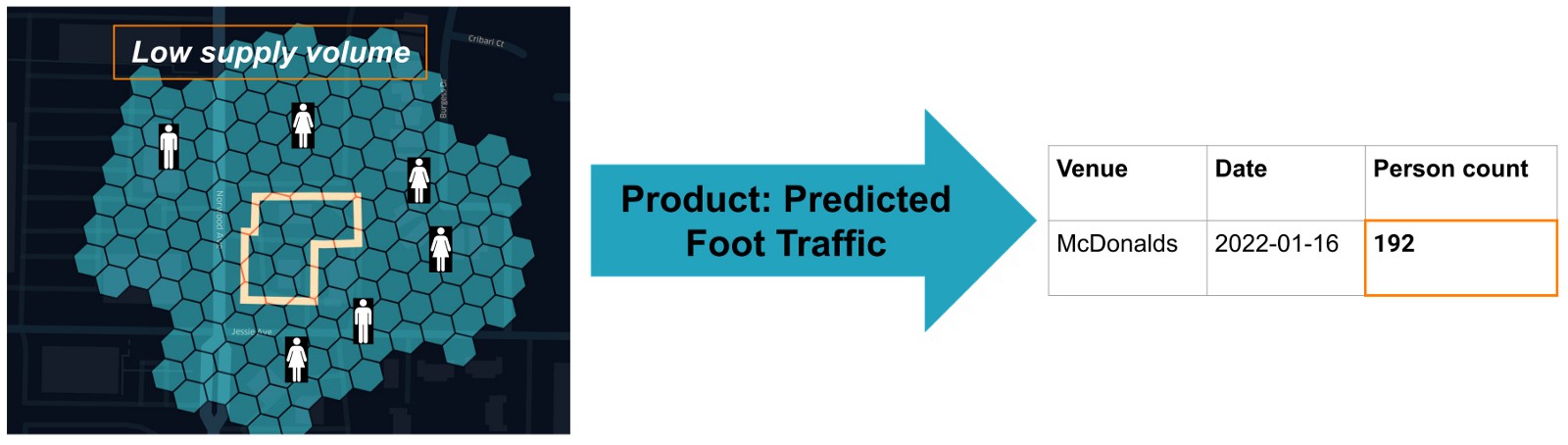
Underlying Contexts in the Model
We use multiple sources of context as features in our machine learning model to estimate the visits. Some of these are:
- Number of people in the vicinity
- Venue square footage
- Local demographics (such as income)
- Holidays
- Day of the week
- Historical data
- People at a location
In total, the model comprises more than 150 features to train those relationships based on our long history of high-quality location data.